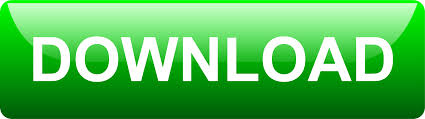

jdbc(url:String,table:String,columnName:String,lowerBound:Long,upperBound:Long,numPartitions:Int.) takes the name of a numeric column ( columnName), two range endpoints ( lowerBound, upperBound) and a target numPartitions and generates Spark tasks by evenly splitting the specified range into numPartitions tasks.You can use two DataFrameReader APIs to specify partitioning: See the Spark SQL programming guide for other parameters, such as fetchsize, that can help with performance. Each task is spread across the executors, which can increase the parallelism of the reads and writes through the JDBC interface. In the Spark UI, you can see that the number of partitions dictate the number of tasks that are launched. Consider database-specific tuning techniques.Consider whether the number of partitions is appropriate.Determine whether the JDBC read is occurring in parallel.Read from JDBC connections across multiple workers.Push down a query to the database engine.Connect to a PostgreSQL database over SSL.Step 3: Check connectivity to the SQLServer database.Step 3: Check connectivity to the MySQL database.Step 1: Check that the JDBC driver is available.Introduction to importing, reading, and modifying data.Databricks Data Science & Engineering guide.
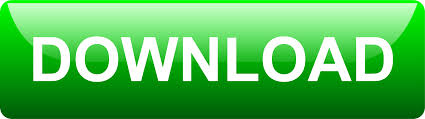